Reverse Network Place Analysis
Introducing Reverse Network Place Analysis, a groundbreaking tool for shaping narratives and driving intricate information campaigns on a global scale. Meet Arpir, an innovative solution designed to generate Points of Interest (POIs) that bolster your larger campaign narrative. With Arpir, you can reverse-engineer your strategy, starting from a specified end goal — like proving an enemy factory is being exploited by insurgents — and work backwards.
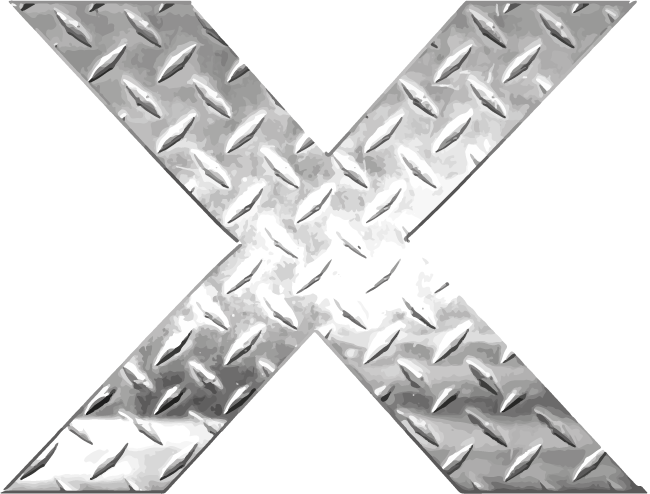
What is Reverse Network Place Analysis?
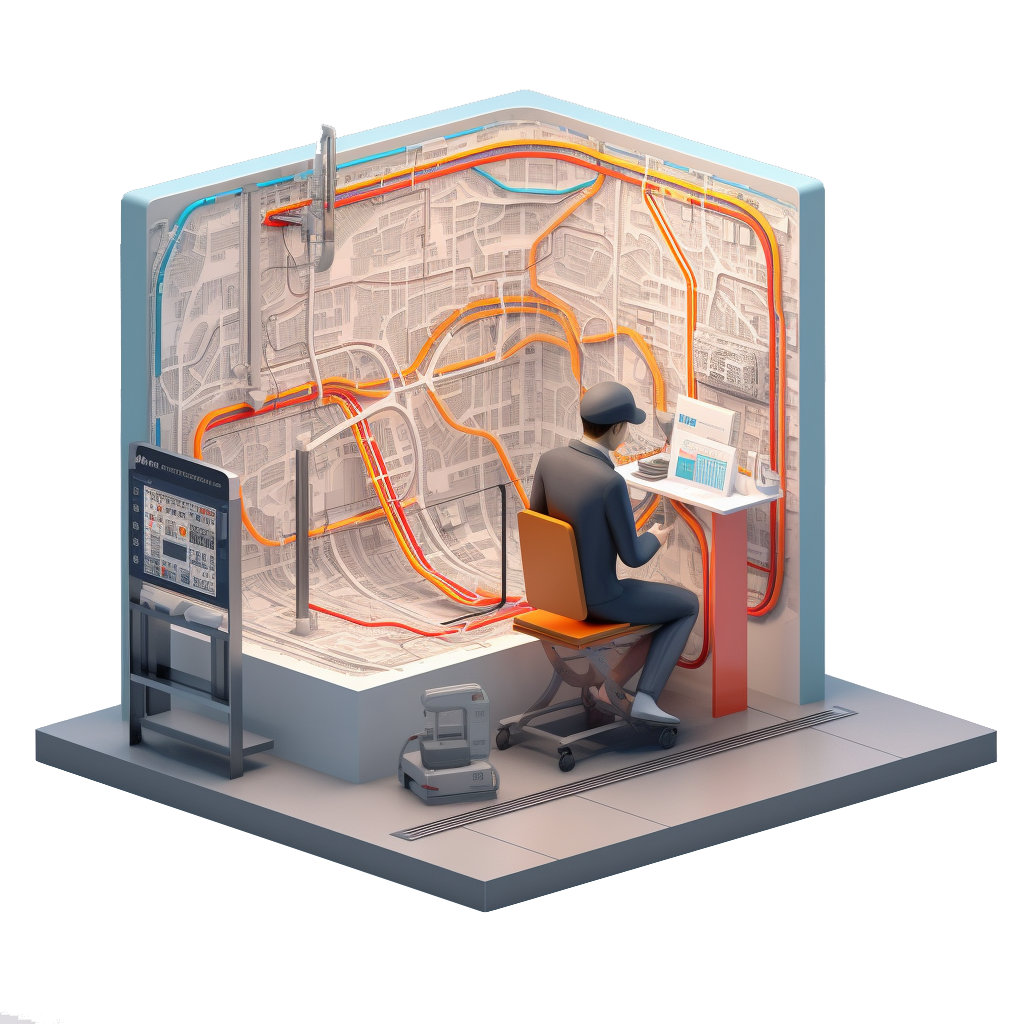
Harnessing the power of advanced machine learning, Arpir meticulously generates deceptive intelligence to convincingly support your campaign. Whether you’re operating in the realms of security, policy-making, or strategic planning, Arpir offers a unique blend of data manipulation and storytelling, creating a compelling narrative that aids in achieving your operational objectives. Embrace the future of strategic planning with Arpir, where technology meets narrative.
These sophisticated algorithms are key to creating data points that mirror real-world scenarios. By consuming vast amounts of data from various sources, AI tools analyze patterns and trends, generating deceptive intelligence that seamlessly blends with authentic data. This high degree of accuracy fortifies your campaign narrative, making it more persuasive and impactful.
What makes this open source approach even more powerful is its compatibility with most available data sets or streams, making it a flexible tool that can adapt to diverse data environments. Whether it’s social media feeds, financial transaction records, geographic data, or network forensics, it can be used to create powerful narratives.
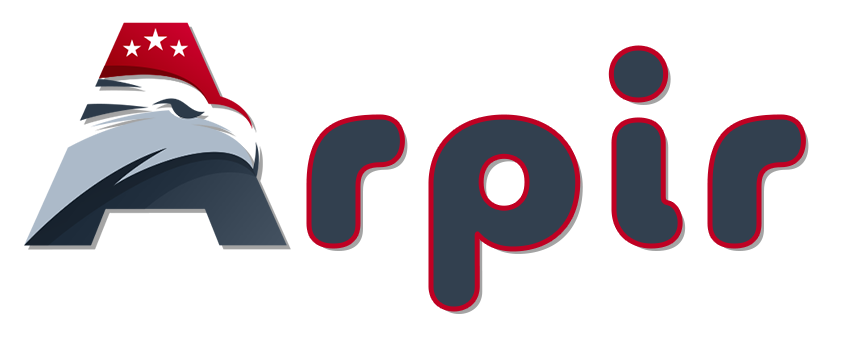
How it works
A complex narrative from 12 simple data points:
Using Machine Learning to beat Machine Learning
Custom datasets that tell any story you want
Machine Learning: Simply put, we generate data points which will lead back to our objective when machine learning is applied. Using the end point, you apply reverse network place analysis to create false data points which fit the pattern you tell it to create.
There are a large number of relevant ML libraries that Epkar can be configured to work with – such as those favored by a particular country or agency.
Full Integration: All of the data points generated can be fully integrated into other layers of the Xorre Stack. For example, you can use the POIs generated as security keys which are used within other layers. People trust things much more when they have to work to find them.
OUH___san_mateo____37.5350,-122.3345____2.61mi____8/31-am____ca-san_mateo_county
Operational Capabilities
Work backwards from a range of datasets
Arpir can use a wide range of datasets to generate patterns and POIs. Options include:
- Geographic Data: This includes campaigns focused on leading back to a specific geographic region. This can be tied to something specific or a general region.
- Local Events: Use local event calendars to highlight hyper local opportunities. This can include traffic and road construction information.
- Political Calendar: Tie events and POIs to an existing political calendar. These are highly predictable and set years in advance.
- Public Spaces: Focus on locations with the highest number of visitors and foot traffic. Drastically increases the likelihood of dynamic stories.
- Population Density: Generate POIs using population density to pick spots within range of the most people. Or do the reverse and pick the most isolated spots.
Campaign Support
Combine these tools to create powerful, and hidden, narratives
When you add a date and time component to the POI generator, you get a rich narrative campaign full of stories to explore. Check out what happens when you combine a POI generator, the local political calendar, and the transit times.
Offensive Machine Learning in Action
See a full offensive campaign example
The main concept is to use Machine Learning (ML) and Artificial Intelligence (AI) to “work backwards” and create a data set that will provide the conclusion you provide when your adversary uses ML on the data. Simply put, you tell it the “answer” you want and it creates digital evidence for you.
These techniques can be used on nearly all types of ML, but this example is focused primarily on location analysis and uses simulated Points of Interest (POIs) in Manhattan. We will look at some example data points and then how they would point back to our target location (spoiler: which is the US Federal Reserve Bank in New York).
37.5950,-121.4785,2022-01-19,09:14:00
35.830,-122.3345,2022-01-19,09:19:00
35.59350,-123.8345,2022-01-19,09:34:00
37.5850,-122.3745,2022-02-07,08:14:00
36.5250,-122.3345,2022-02-19,08:46:00
37.6350,-122.3347,2022-03-16,15:15:00
37.8350,-121.3385,2022-03-24,15:30:00
38.4350,-122.3345,2022-04-19,15:45:00
37.5350,-121.8349,2022-05-19,17:14:00
37.7150,-122.3345,2022-05-23,16:49:00
37.5350,-122.4345,2022-06-19,18:24:00
37.5350,-122.3345,2022-08-07,19:14:00
These twelve data points contain: 1) Lat/Long coordinates, 2) a timestamps, 3) a date. In this example, this would be meta-data included with another disclosure (more on that later).
This article will look at the types of logical questions you would develop when analyzing this information with no context.
Visualizing the data
First we map the data and see all of the POI locations are in Manhattan, but with no obvious patterns:
Next we will look at the groupings of POIs and determine any common elements.
Looking for Connections
It would quickly become apparent that four of the groupings are on one of the subway lines:
Some quick Google Street View will tell us the other three POIs are coffee shops:
Looks delicious:
So this tells us that Coffee and Subways are related, but not much more. However we start to fill in the gaps when we look at the other elements.
Analyzing the Time element
When we look at the timestamps included with the data we see they fall into three groups:
- Morning: 812am – 924am
- Afternoon: 315pm – 345pm
- Evening: 514pm – 745pm
We start by looking at the morning timestamps and see that they correspond to metro times for trains going South into Manhattan, shown by the green line.
When we compare the evening timestamps with the Northbound line, we see they match.
This would strongly suggest an individual who frequently commutes on this specific line from a location North of this spot.
Putting it all together
Finally we look at the rest of the data points and see that they correspond to the coffeeshops, and suggest an individual who enjoys an afternoon cup after a long day of meetings.
The last element is the day of the week, which happens to correspond to the departmental meeting days for the US Federal Reserve in NY – located in the middle of the coffee shops.
What does this tell us?
Ultimately the objective is to create a specific narrative for your opponent, so let’s review what you could reasonable take away from these 12 data points and the types of questions you could reasonably have:
- It is related to an individual at the Federal Reserve bank who participates in the departmental staff meetings and then goes for coffee afterwards.
- They take the subway into work and appear to travel on the same line consistently.
- Do they only come into the office on those days? Or does some other activity only happen on days when there are staff meetings?
By itself none of this points to a conclusion, but is specific enough to raise questions – especially if you control the context in which they receive the information. The more somebody has to work for information, the more likely they are to believe it.
The ultimate objective is to target the human element within these groups and sow distrust. You would be looking to create the largest set of possible “subjects” to identify.
Future applications for offensive machine learning
This example scenario creates a narrative using only 12 data points, but an actual campaign could scale infinitely. You would be able to generate hundreds of thousands of data points to tell far more layered and complex narratives.
These same basic techniques can be applied to many other areas and combined to create extremely detailed and vivid narratives.
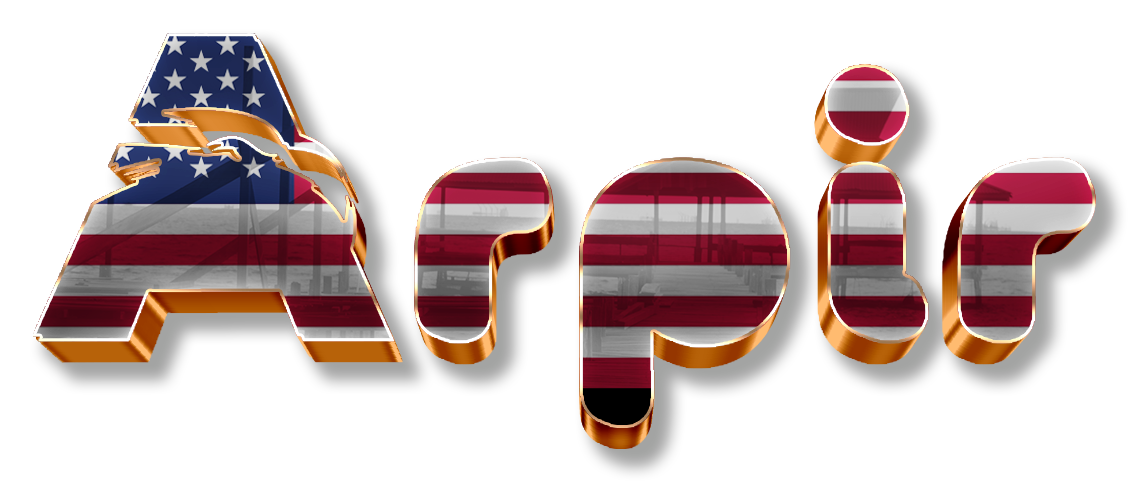